MIT Researcher: Artificial Intelligence Has a Race Problem, and We Need to Fix It
The next generation of AI is poisoned with bias against dark skin, Joy Buolamwini says.
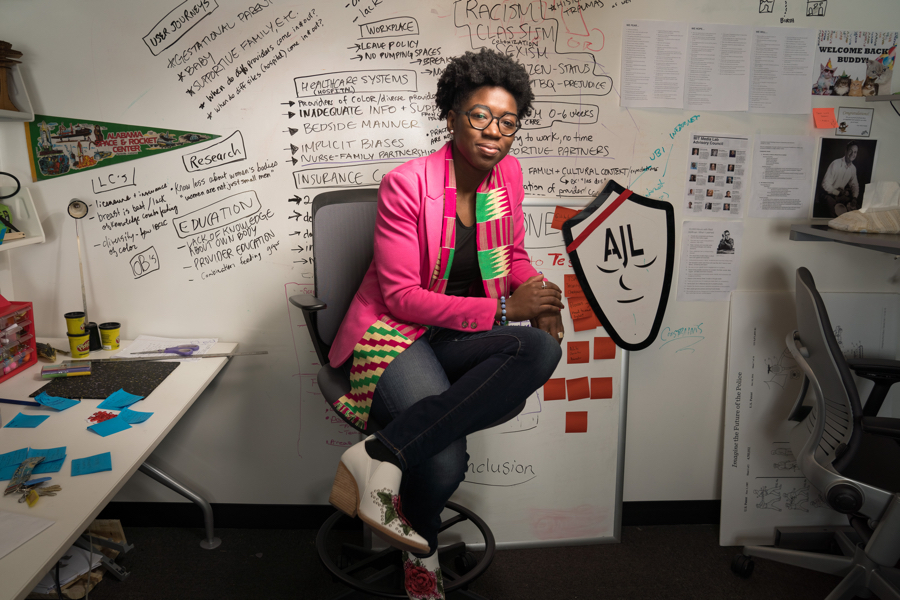
photo by Bryce Vickmark
Artificial intelligence is increasingly affecting our lives in ways most of us haven’t even thought about. Even if we don’t have emotional androids plotting revenge on humankind (yet), we’re surrounded more and more by computers trained to look us over and make life-changing decisions about us. Some of the brightest minds in technology—including a hive of them clustered around Boston—are tinkering with machines designed to decide what kinds of ads we see, whether we get flagged by the police, whether we get a job, or even how long we spend behind bars.
But they have a very big problem: Many of these systems don’t work properly, or at all, for people with dark skin.
In a newly published report, MIT Media Lab researcher Joy Buolamwini says she’s found that face-analyzing AI works significantly better for white faces than black ones. She and co-author Timnit Gebru tested software from Microsoft, IBM, and the Chinese company Megvii to see how well each of their face-scanning systems did at figuring out whether a person in a picture was a man or a woman—a task all three are supposed to be able to perform. If the person in the photo was a white man, her study found, the systems guessed correctly more than 99 percent of the time. For black women, though, the systems messed up between 20 and 34 percent of the time—which, if you consider that guessing at random would mean being right 50 percent of the time, means they came close to not working at all.
How could that be? Buolamwini says the problem lies out of sight, buried deep in the code that runs this technology. Face-analysis programs, she says, are trained and tested using databases of hundreds of pictures, which research has found are overwhelmingly white and male. So a developer might not notice that their software doesn’t work for someone who isn’t white or male. To prove this, Buolamwini decided to test the systems by using a database of her own, which she made using the headshots of hundreds of world leaders, including many from African countries with dark skin. Then she asked the AI systems to identify whether each headshot was of a man or a woman. That’s when the disparity began to show itself: The systems repeatedly misidentified the black faces, even though they had almost no trouble at all recognizing the white ones. Not that it’s exactly a surprise. “Being that I’m a dark-skinned woman,” she tells me, “I’m running into these issues all over the place.”
The findings were compiled into a project called Gender Shades and will be presented at the Conference on Fairness, Accountability, and Transparency at New York University, which kicks off on Friday.
We should all be concerned about what she found.
“People of color are in fact the global majority. The majority of the world, who I like to call the under-sampled majority, aren’t being represented within the training data or the benchmark data used to validate artificial intelligence systems,” Buolamwini says. “We’re fooling ourselves about how much progress we’ve made.”
Imagine if your skin tone could trigger glitches in services like HireVue, which analyzes the facial expressions of job applicants; or in surveillance systems that use AI to identify people in crowds (like the one used on unwitting Boston Calling concertgoers in 2014); or the face-recognition system currently being test-run at Logan Airport; or in the systems used by law enforcement to trawl through Massachusetts drivers’ license photos.
Frankly, it’s not clear whether any of these systems should exist at all. How good do we really want robots to be at tracking our every move? But at the very least, if these things are going to be part of our lives, they’d better work. If not, the consequences could be severe.
“You’re in a situation where the community most likely to be targeted by law enforcement are least represented [in face-recognition code]. This puts people at higher risk of being misidentified as a criminal suspect,” Buolamwini says. “Because we live in a society that reflects historical biases that are continuing to this day, we have to confront the kind of data we’re generating, the kind of data we’re collecting, how we’re analyzing it. And we need to do it with diverse eyes in the room, diverse experiences, and more gender parity.”
Last year, the ACLU identified “algorithmic bias” as an area of particular concern for civil liberties advocates in the future. “This is not remotely the first time that we’ve seen a product developed using machine learning or a risk assessment tool that has different impacts on people, depending on who they are: their race, their class,” says Kade Crockford, director of the ACLU of Massachusetts’ Technology for Liberty program. “Civil rights, civil liberties, and freedom of speech, and privacy tend to be afterthoughts when systems like this are being developed. What we really need to see is an ethical, civil rights and civil liberties approach to building these systems from the ground up.” The group’s local chapter has also pushed for Community Control of Police Surveillance ordinances in Massachusetts cities that would require that any new surveillance tools get public approval before they are implemented.
There are other impacts of technology that only works correctly for white people. By now you’ve probably seen the videos and heard the stories about soap dispensers that won’t dispense soap into dark-skinned hands, or artificial intelligence that tries to identify African-American faces and yields some deeply unfortunate results. Examples of this problem also predate AI, Buolamwini says. Photography for most of its history has been optimized for white skin. It wan’t until backlash from manufacturers of chocolate and dark wooden furniture in the 1970s and 1980s that Kodak, which basically ran the photography industry, updated its film, and we’re still making progress on this front in the era of digital cameras and selfies (just ask interracial couples). Movies with properly lit black actors are still a relatively new phenomenon.
Buolamwini knows this problem well. She still remembers when, as an undergrad at Georgia Tech, she couldn’t complete her work with a new face-tracking robot because it couldn’t make sense of her face. She was forced to ask her light-skinned roommate, who the robot could see, to step in. It wasn’t the last time this happened, and eventually she made the disheartening discovery that many face-recognizing systems would only work if she wore a featureless white mask. The poetry of that wasn’t lost on her, and she’s turned that mask into a symbol of what she’s dubbed the “coded gaze” baked into AI. The mask was a centerpiece of her popular TED Talk (which has been viewed nearly a million times) and is featured in the logo for a group she founded to call attention to the issue, the Algorithmic Justice League (you can see it next to her in the above photo).
Meanwhile, Buolamwini says she’s been encouraged by some of the reactions her study has gotten. IBM, she says, reached out almost immediately after her results were published and invited her to meet with some of its senior researchers. The tech giant tells her that in the newest update of its software, it improved its ability to recognize black women’s faces by a factor of 10. Microsoft has also responded to the Gender Shades research, saying it has “taken steps to improve the accuracy of our facial recognition technology and we’re continuing to invest in research to recognize, understand and remove bias.”
There’s a long way to go. So she’s focused on getting us to think twice about what’s going on in the rapidly advancing brains that power artificial intelligence, before it’s too late.
“We have blind faith in these systems,” she says. “We risk perpetuating inequality in the guise of machine neutrality if we’re not paying attention.”
Correction: An earlier version of this story misidentified the college Buolamwini attended for her undergraduate degree. It was Georgia Tech, not MIT.